A siren is a loud noise-making device mounted in fixed locations and used to warn
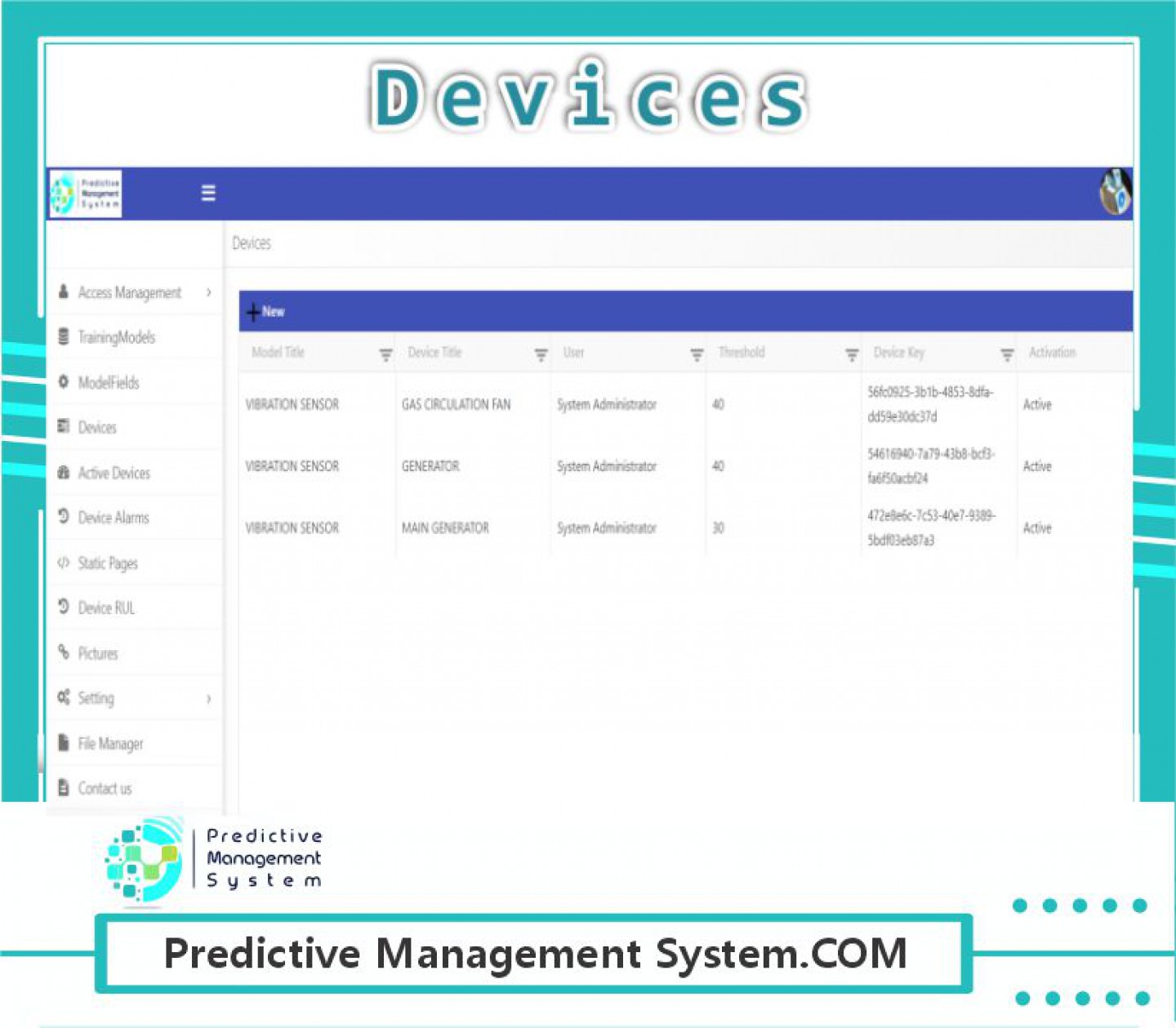
In various programs, there are various formats to show
The forth way we reduce environment
Secondly, using our software, we increase the
Predictive Management Services Co.
The Company will be incorporated in the Province of Manitoba as a numbered corporation. It will be owned and managed by Mr. Reza Abbasi, Mr. Mehdi Motahari, Mr. Hassan Rezaei, Mr. Siamak Soltanian, and Mr.Abdolreza Khamenhei.
Predictive maintenance (PdM) is a type of condition-based maintenance that monitors the condition of assets using sensor devices. These sensor devices supply data in real-time, which is used to predict when the asset will require maintenance and prevent equipment failure.
Implementation a software based on Machine Learning (ML) and Artificial Intelligence (AI) in order to:
• Save taxpayer dollars from wasteful spending on reactive maintenance also known as
breakdown maintenance to provide an efficient and cost-effective solution
• Partner with B2B Companies to establish an ongoing win/win strategy
• Implement value-based pricing to incentivize the staff
• Steel Production
•Petrochemical Industry
•Chemical Industry
•Automotive Industry
Our vision is to connect things, apps, and people together for working smarter and more efficiently.
Working with valuable assets to try to increase the life of the assets and decrease the degradation period through innovation and customization and provide solutions through automation.
• An innovative Predictive Maintenance (PdM) procedure is proposed. In this novel technique, sensors are connected to the system's operating parts, and some parameter values (such as pressure, vibrations, temperature) are always being measured and recorded for each system element by IoT technology. The data recording process is initially done at the plant, and the sensory data is collected in various operational situations such as optimal and sub-optimal instances. The learning type is supervised learning, and these sensory data are labeled with their working conditions. Before the teaching/training process begins, a preprocessing of raw sensory data is necessary, which includes repairing the missing data, noise reduction, encoding categorical data, separating training and test sets, and feature scaling. A server should be placed in the plant, which is responsible for data preprocessing. The preprocessed data is sent to a cloud server which hosts the trained model.
• The recorded information is used to train a machine learning model; in this case, the Artificial Neural Networks (ANN); which is used as a Digital Twin (DT). ANN is used to classify the outcome and be utilized for predicting the fault type and predicting the breakdown time. A time-series method is used within an ANN that is capable of predicting recurrence. ML algorithms are applied to distinguish hidden correlations in data sets and detect abnormal data patterns. A Graphical User Interface (G.U.I.) is connected to cloud service, and the maintenance staff can use the G.U.I monitor the system condition, and as soon as a system element is predicted to be on the verge of failure, G.U.I warn the staff, and they can immediately replace the component.
• The D.T. can predict the time and type of break down before it happens. Therefore, it can prevent both system failures and early part replacements. It helps to avoid production rate reduction as well as unnecessary replacement costs. The trained model's performance is gradually boosted as more data is logged in the system. Besides, different plants can share their data on the cloud to help each other's D.T.s quality by increasing the data volume. Models provide the information on how many cycles are left until a machine's useful life ends. Predictive models are regularly updated, depending on the industry, at least every month, and tested for accuracy. If there are variances between the outputs from the expected one, they are investigated, revised, retrained, and tested again.
1600 Amphitheatre Parkway, Mountain View, CA 94043
+1 650-253-0000
prothemes.net@gmail.com
Daily: 9:00 am - 6:00 pm
Sunday: Closed
Recent Comments